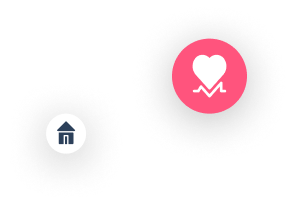
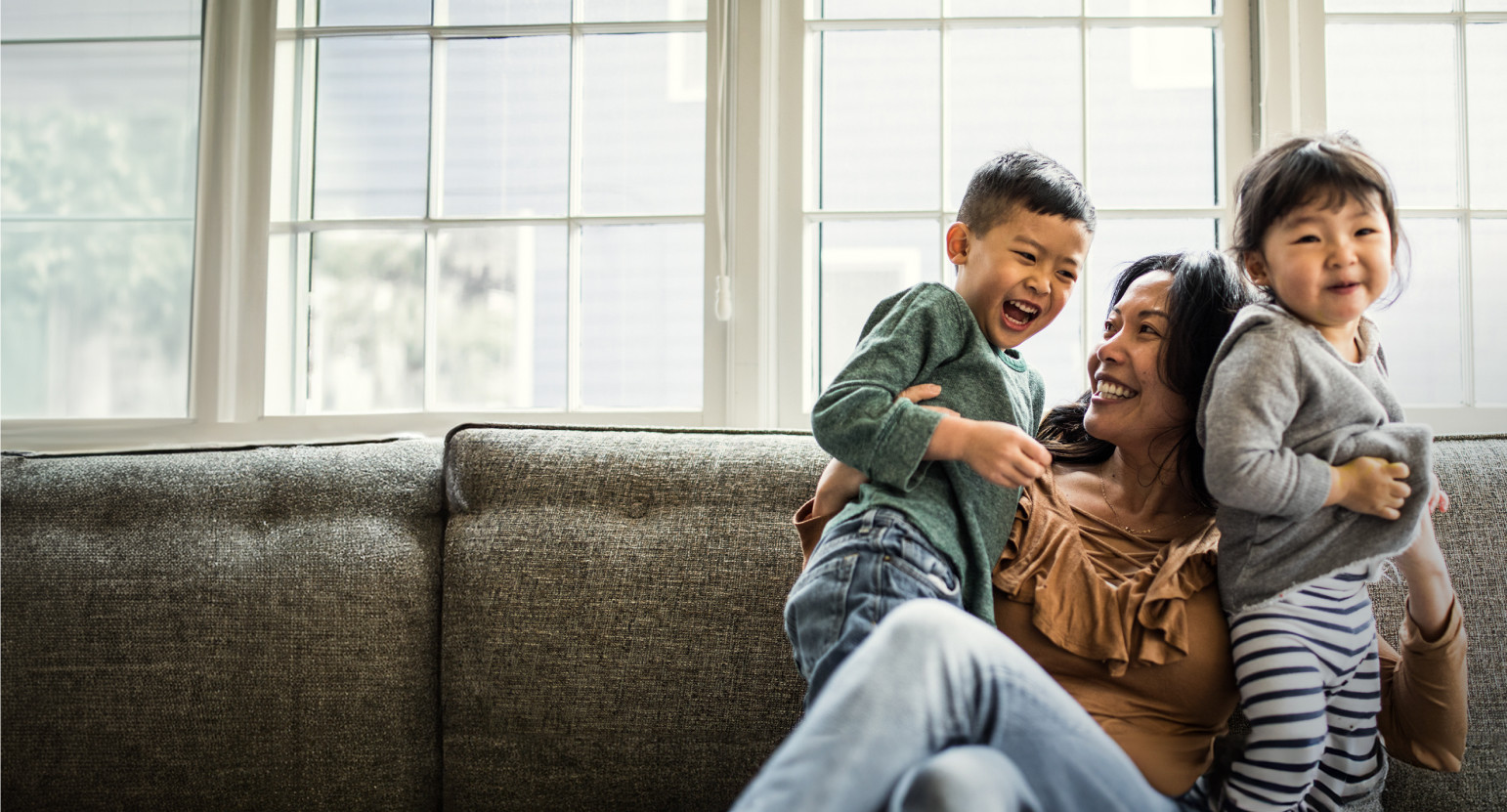
Your Partner
for Social Care
Thousands of government, healthcare, community-based organizations, agencies, and institutions of all sizes partner with Unite Us because of our robust technology, expansive network, and tenured people who support whole-person care.
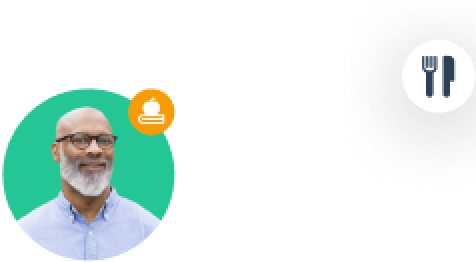

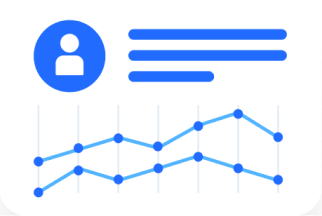
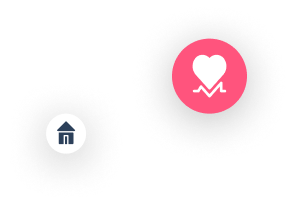
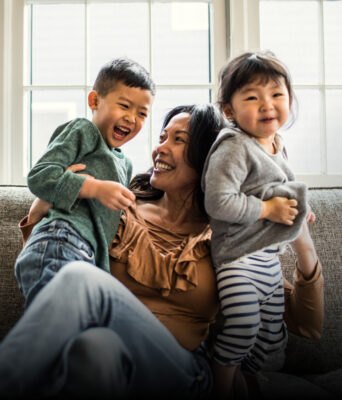

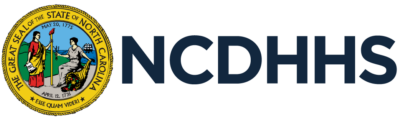
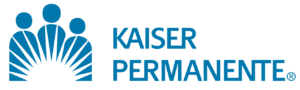
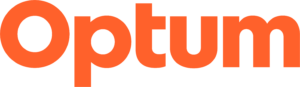
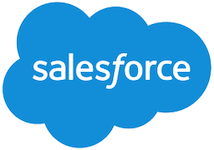
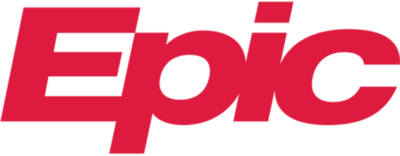
An Integrated Suite of
Social Care Solutions
Reduce costs, streamline processes, and support your clients more efficiently with one platform. Use Unite Us to coordinate closed-loop referrals and share trusted resources securely, Insights to inform your decision-making with comprehensive social care data, Payments to help you seamlessly manage social care funding at scale, and Care Coordination to ensure needs are addressed with our dedicated, professional support and expertise.
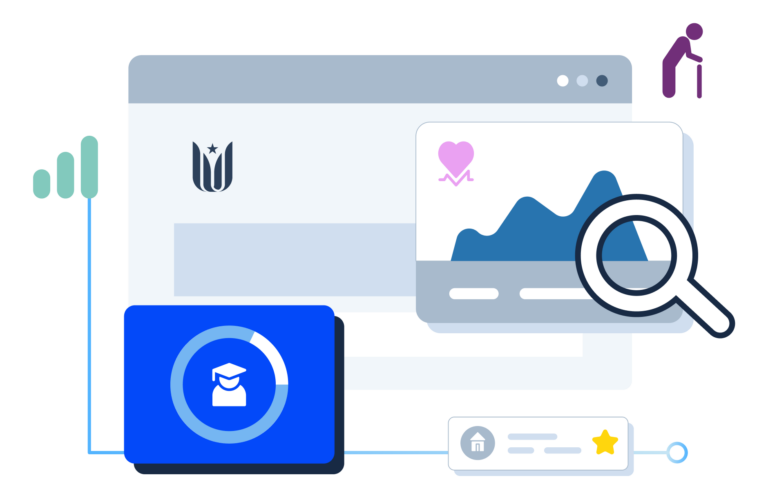
Meeting You Where You Are
Every community is different. Every case is unique. Unite Us makes it possible to customize care solutions to the needs of every individual. Our network and teams span across the country, supporting organizations with the flexibility and resources you need when you need them.
1000+
33M
1M+
740+
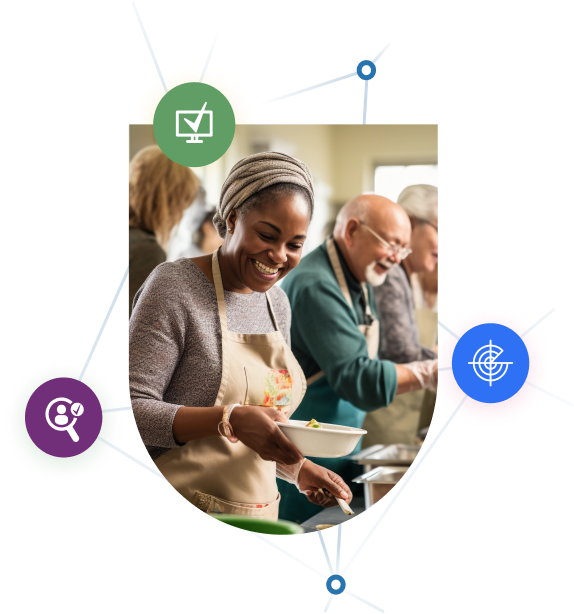
Improving Health and Well-Being is Our Goal
You can use Unite Us software to speed up the delivery of referrals and reach outcomes faster. Get expert advice from our Care Coordination and Research and Evaluation teams and see value with Unite Us faster.
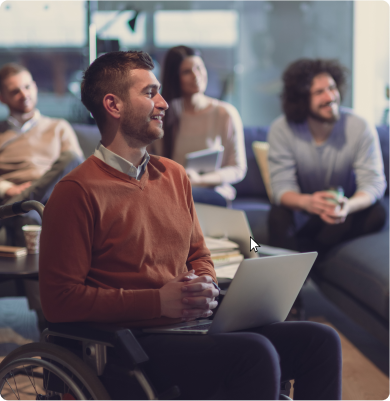
Technology
Automate your community management and coordination programs with one platform.
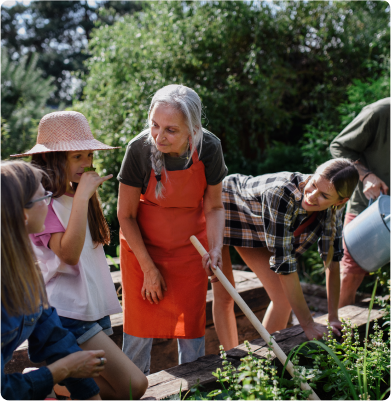
Network
Build community connections, understand population needs, and address gaps.
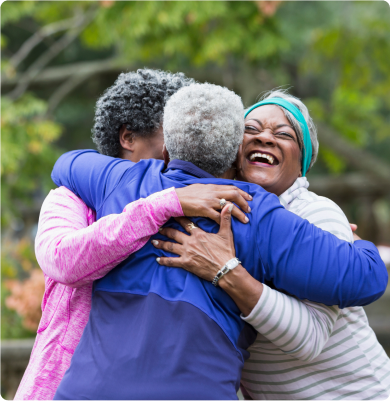
People
Leverage our expertise to deliver individualized care, wherever you are on your journey.
It’s Easy as 1-2-3
Our implementation process is divided into three distinct phases for easy comprehension and execution. Once onboarded, our Customer Success team will meet with you regularly to review network health KPIs and track overall progress.
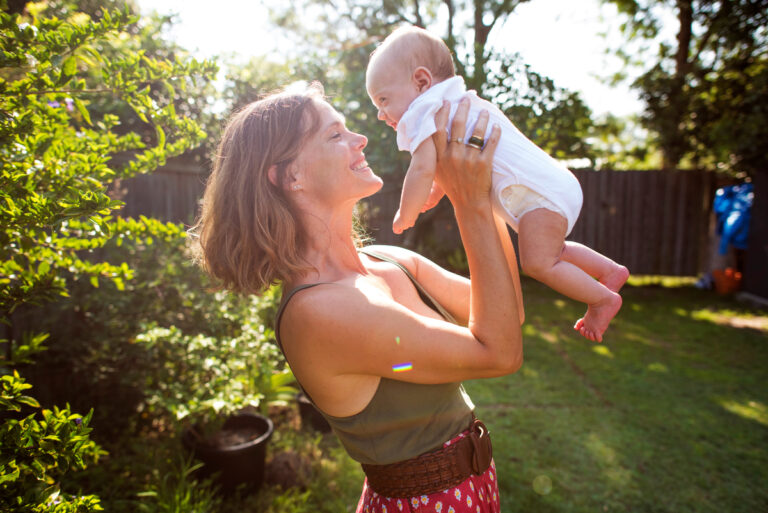
How Social Care Improves Maternal Health Outcomes: Learn from Innovators at Sarasota Memorial Health Care System
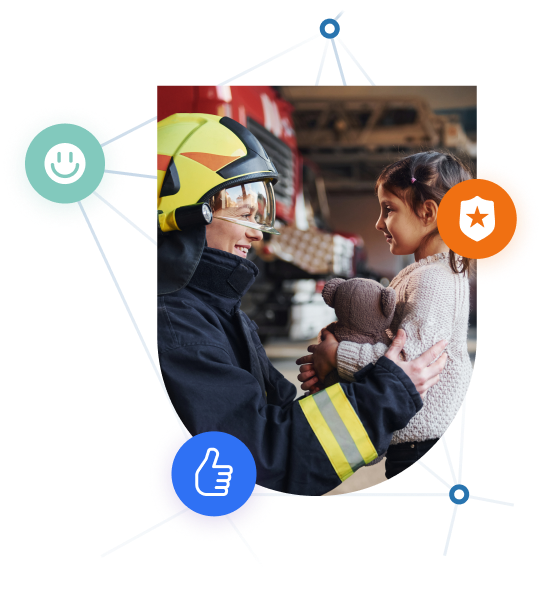
Start building healthier communities today.
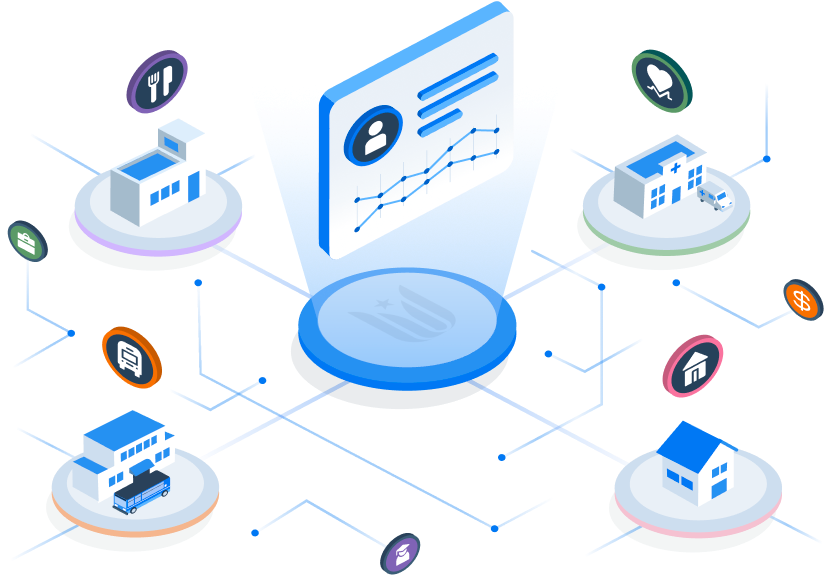